DSPM vs Traditional Data Discovery Tools

The amount of data generated, used and stored will reach 180 zettabytes by 2025. This amount is staggering, especially given the fact that 59% of security leaders say they struggle to maintain a view of their data.
Can you discover and classify a growing data estate with manual tools and processes?
In a survey with security leaders, the answer is likely no. 47% say that existing manual processes for data security are cumbersome while 39% say that legacy technology is insufficient for current requirements.
Traditional data discovery and classification technologies that rely on manually-configured connectors, rule-based classification, and human-initiated efforts are a significant mismatch for the staggering volumes and variety of data that organizations hold.
Below, we look at why it's time to leave traditional data discovery tools in the past and why it’s time to adopt Data Security Posture Management (DSPM).
7 Problems with Traditional Data Discovery and Classification Tools
When we talk about "traditional data discovery tools," we refer to data discovery and classification technology that require manual connections to datastores, use slow scanning processes, and rely on rule-based classification, taking months to years to implement.
These are the kinds of “smart data discovery tools” that came to market in the mid-2010s. They lack the ability to keep up in the cloud era, where data is constantly being created, copied, and moved. By the time a traditional tool finishes scanning a datastore, the data has already changed several times over.
Unfortunately, many of today’s technologies have built-in, data discovery and classification functionality that use this traditional approach, even though they are marketed as “automated.” These technologies include DLP (Data Loss Prevention), data privacy, data catalog, legacy data security, and even Cloud Security Posture Management (CSPM).
Specifically, these traditional data discovery and classification fail modern security challenges because of the following:
1. Incomplete findings
Traditional tools have limited ability to discover different types of data.
These tools are not designed to uncover ‘unknown unknowns.’ Admins operating the tools must list out the datastores they plan to scan and obtain access credentials for each one. When admins are not aware of the datastore, then that datastore will not be scanned and the data within will not be discovered.
Some of these tools are designed for one type of data, such as unstructured data but not structured. Therefore, teams that rely on these tools may find themselves without visibility to 20% of the data in their data ecosystem.
Traditional tools are not able to make sense of data that is unique to a business. These types of data do not conform to a specific, predefined pattern that these tools use to identify data. For example, “employee ID” can be unique and different across organizations. Some may utilize 10 digits for this number, others may add in a mix of symbols, numbers, and letters, all of which make it harder to be understood by static rules.
2. Prolonged and technical implementation processes
Implementation can involve installing agents and manually configuring a mix of hardware and software, depending on the location of data. This can mean months of deployment and configuration time before seeing any value.
Add to this the fact that connector quality may vary across sources. In order to conduct a scan for certain sources, admins must bring in developers to establish a connection. This means more time and resources required to initiate the scanning process.
3. Time-consuming and inaccurate manual classification
Traditional data classification processes rely on regular expressions (Regex) to label data. Regex requires specialists to spend significant time building rules, then validating them. It’s not uncommon for organizations to have dedicated specialists, building regex rules. These specialists can spend weeks constructing dozens of regex rules for a single classifier.
The hundreds of predefined classifiers that these technologies push forward may save you time from building your own rules, but they yield widely inaccurate outputs. It’s not uncommon for teams to adopt these predefined classifiers, only to realize that the majority of outputs are wrong and require human validation.
Some technologies require users to manually label data. Any labeling system that is manually applied is at best reflective of a point-in-time, and at worst doomed to lead to false negatives that lead to breaches.
4. Lack of scalability
The performance limits of traditional tools mean they can take days to scan a single datastore unless they cap scan sizes. However, this will only work when the scanned datastore is small and has uniformly structured data. A tool that uses capped scans to analyze larger or more complex datastores with unstructured data will end up providing unreliable results. This is not an approach that will scale with the increase in data volumes.
5. Lack of context into risks
Traditional tools don't fill the information gap that security teams need to manage risks—understanding the context that expose data to increased security and privacy risks.
Context about the security of the data tells you if confidential, restricted, or otherwise sensitive data is exposed as plaintext, violating data security or privacy rules. Context about the data subject role of the data informs you if it’s about a customer or employee, so you know how to handle that data. Context can also tell you if the data belongs to a resident of e.g. Germany, so you can adhere to GDPR’s data sovereignty requirements. Many traditional tools claim that cloud metadata or tags provide context, but when metadata and tags are manually applied or derived from pattern matching, the understanding of data is based on assumptions that lead teams astray.
6. Outdated views
The rule-based classification processes that traditional tools use only give static snapshots of your data. As data changes, that view remains the same. This means you only possess stale views of data, even when the risk levels change pertaining to that data.
When data discovery or classification is not able to identify changes to the data, organizations are not able to apply the most appropriate controls to the data, for example, limiting access or encrypting data at rest.
7. High operational costs
Technologies that rely on traditional data discovery can quickly run up costs just for using the tool. To gain an accurate understanding of the data, these tools require scanning large volumes of data. When vendors charge by volume, this can make scanning costs unpredictable, causing misalignment between security and cost control goals.
The DSPM Advantage
Data Security Posture Management is the automated alternative to traditional tools. Cyera delivers an AI-powered platform with DSPM to help you understand and secure your sensitive data.
Cyera’s approach has several advantages over traditional technology by:
- Connecting to datastores through cloud-native APIs, without having to rely on agents
- Identifying diverse data types including structured and unstructured data, known and unknown data, and unique data classes
- Covering data across different on-prem and cloud service models (IaaS, SaaS, PaaS/DBaaS)
- Delivering value in days and weeks, instead of months and years
- Providing continuous visibility and dynamic identification of data changes
- Ensuring highly accurate classification through AI-powered technology
- Enriching data understanding with context about what the data represents
DSPM Versus Traditional Data Discovery and Classification Tools
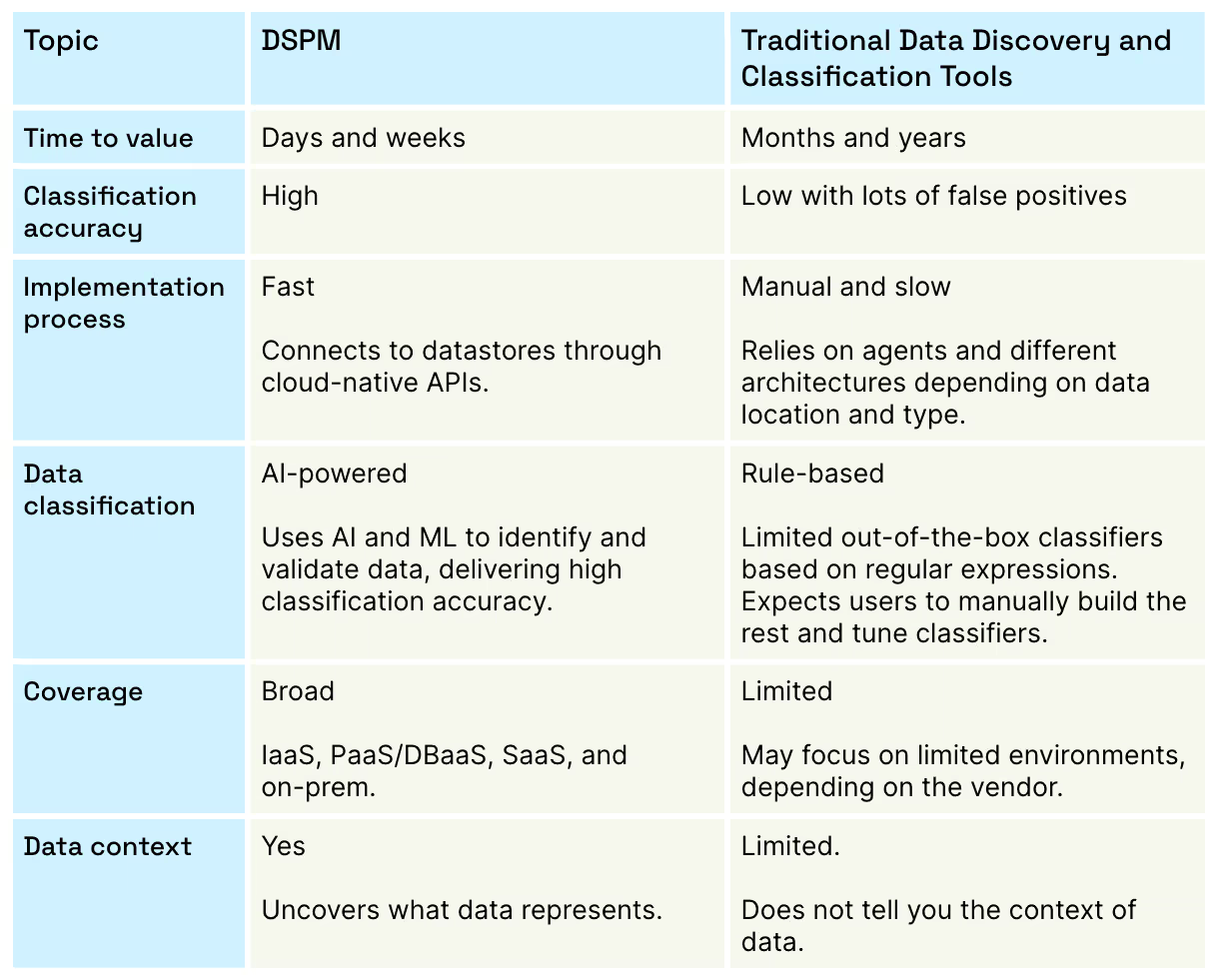
Can Your Traditional Solution Meet These 9 Challenges?
In another blog, we dive into the capabilities that organizations now need to have to prevent and respond to data exposures. If you are already using a traditional data tool, it's worth asking yourself whether it can:
- Automatically discover your multi-cloud and on-prem data without agents.
- Give you instant results, even in places or for data you didn’t know you had.
- Show you context about data, like where the data subject resides (i.e., do they fall under the GDPR or the CCPA) and whether the data is synthetic.
- Show risks of PII exposure, based on the combination and proximity of data.
- Provide a single source of truth for data oversight for security teams.
- Show intelligent insights through AI, like why a user has access to certain data.
- Give you the information you need for data security or compliance issues prioritization.
- Find known and unknown data.
- Scale with your data growth to scan petabytes of data, quickly.
If the answer to any of these questions is negative, it's time to schedule a demo and see how a cloud-native DSPM from Cyera can provide you with the data visibility you need to secure your data.
Gain full visibility
with our Data Risk Assessment.